Episodes
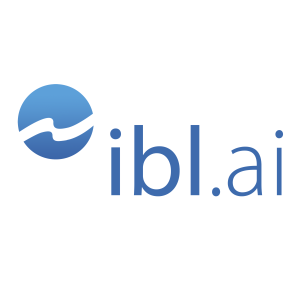
Wednesday Nov 06, 2024
Wednesday Nov 06, 2024
Summary of https://conference.nber.org/conf_papers/f210475.pdf
This research paper examines the impact of an artificial intelligence (AI) tool on materials discovery in the R&D lab of a large U.S. firm.
The AI tool, which leverages deep learning to partially automate the materials discovery process, was rolled out to scientists in three waves, allowing the researchers to analyze the effects of the technology. The study found that the AI tool significantly accelerated materials discovery, resulting in an increase in patent filings and product prototypes, particularly for scientists with strong initial productivity.
However, the tool's effectiveness depended on the scientist's ability to evaluate the AI-generated compounds, highlighting the importance of human judgment in the scientific discovery process.
The paper concludes by exploring the AI tool's impact on scientist job satisfaction and beliefs about artificial intelligence, revealing that while the tool enhances productivity, it also leads to changes in the types of tasks scientists perform, potentially affecting job satisfaction and prompting a need for reskilling.
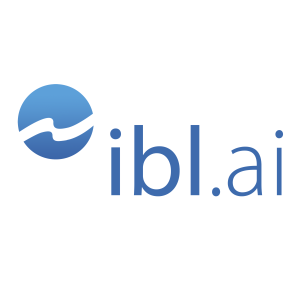
Tuesday Nov 05, 2024
Tuesday Nov 05, 2024
Summary of https://papers.ssrn.com/sol3/papers.cfm?abstract_id=5007084
This working paper investigates the impact of generative AI on the nature of work, specifically focusing on software development. The authors study the introduction of GitHub Copilot, an AI-powered code completion tool, within the open-source software development ecosystem.
They use a natural experiment based on GitHub's "top developer" program, which provides free Copilot access to developers of the most popular repositories. Through a regression discontinuity design, they find that access to Copilot induces developers to allocate more time towards coding activities and less towards project management.
This shift is driven by two mechanisms: an increase in autonomous work and an increase in exploration activities. The authors also find that the effects are greater for developers with lower ability, suggesting that generative AI has the potential to flatten organizational hierarchies and reduce inequality in the knowledge economy.
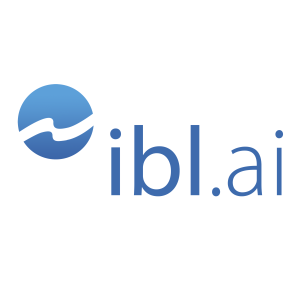
Friday Nov 01, 2024
Friday Nov 01, 2024
Summary of https://www.hbs.edu/ris/Publication%20Files/24-074_bee7fd2f-882e-4e8c-adfe-150f8439dff6.pdf
This working paper examines the challenges of senior professionals learning to use generative AI from junior professionals. The authors argue that while the existing literature on communities of practice suggests that juniors are well-suited to teach seniors about new technologies, this is not the case with generative AI.
The paper highlights the emerging technology risks that senior professionals are concerned about, including inaccurate output, lack of explainability, and the possibility of user complacency. The authors suggest that juniors may recommend ineffective novice AI risk mitigation tactics because of their limited understanding of the technology's capabilities and their tendency to focus on changing human routines instead of system design.
The paper concludes by recommending that corporate leaders should focus on educating both junior and senior employees about AI risks and mitigating these risks through system-level changes and interventions at the ecosystem level.
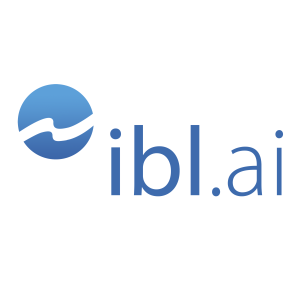
Friday Nov 01, 2024
Friday Nov 01, 2024
Summary of https://arxiv.org/pdf/2410.05229
This research paper investigates the mathematical reasoning capabilities of large language models (LLMs) and finds that their performance is not as robust as previously thought.
The authors introduce a new benchmark called GSM-Symbolic, which generates variations of math problems to assess the models' ability to generalize and handle changes in question structure.
The results show that LLMs struggle to perform true logical reasoning, often exhibiting a high degree of sensitivity to minor changes in input.
The authors also find that LLMs often blindly follow irrelevant information in the questions, suggesting that their reasoning process is more like pattern matching than true conceptual understanding.
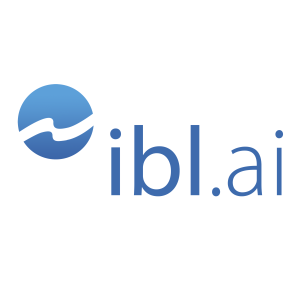
Thursday Oct 31, 2024
Thursday Oct 31, 2024
Summary of https://arxiv.org/pdf/2410.03703
This research paper investigates the impact of large language models (LLMs) on human creativity, specifically focusing on divergent and convergent thinking with over 1,100 participants.
The results showed that while LLMs can provide short-term boosts in performance, they may ultimately hinder independent creative abilities.
The paper concludes that the long-term effects of using LLMs for creativity need to be carefully considered, and AI systems should be designed to enhance, rather than diminish, human cognitive skills.
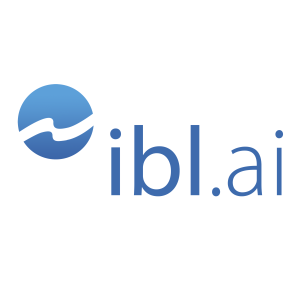
Thursday Oct 31, 2024
Thursday Oct 31, 2024
Summary of https://github.com/ibm-granite/granite-3.0-language-models/blob/main/paper.pdf
This document details the development and release of Granite 3.0, a new family of open-source, lightweight foundation language models from IBM. The paper provides a thorough overview of the models' design, including their architecture, training data, and post-training techniques.
It also explores the models' performance across various benchmarks, focusing on their capabilities in general knowledge, instruction following, function calling, retrieval augmented generation, and cybersecurity.
The paper concludes by discussing the socio-technical harms and risks associated with LLMs and outlines IBM's efforts to mitigate these concerns through responsible AI practices.
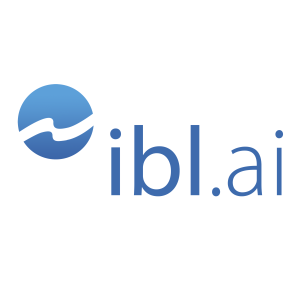
Wednesday Oct 30, 2024
Wednesday Oct 30, 2024
Summary of https://figshare.com/articles/preprint/Generative_AI_for_teaching_and_learning_pilot_project_2024-5_White_paper/27325515/1?file=50061531
This document proposes a project at George Washington University led by Professor Lorena Barba to implement a course-level AI mentor platform, which would allow instructors to create customized AI assistants for their students.
The ibl.ai platform aims to address the growing use of AI among students and to harness the technology's pedagogical potential. The document outlines the platform's features, benefits, deployment plan, research component, and potential vendor options.
It also highlights the importance of building AI literacy among faculty and students.
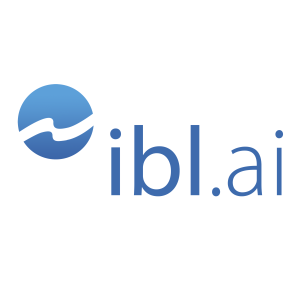
Wednesday Oct 30, 2024
Wednesday Oct 30, 2024
Summary of https://tech.ed.gov/files/2024/07/Designing-for-Education-with-Artificial-Intelligence-An-Essential-Guide-for-Developers.pdf
This guide, produced by the U.S. Department of Education, provides recommendations for developers of Artificial Intelligence (AI)-enabled educational technology (edtech) products.
The guide emphasizes building trust within the edtech ecosystem through five core recommendations: designing for education, providing evidence for rationale and impact, advancing equity and protecting civil rights, ensuring safety and security, and promoting transparency and earning trust.
The guide emphasizes shared responsibility between developers and educators to create and deploy AI in education in a safe, secure, ethical, and equitable manner, while aligning with existing federal regulations and best practices.
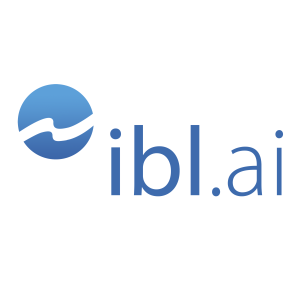
Monday Oct 28, 2024
Monday Oct 28, 2024
Summary of https://ai.wharton.upenn.edu/wp-content/uploads/2024/10/AI-Report_Full-Report.pdf
"Growing Up: Navigating Gen AI's Early Years", is a report from AI at Wharton and GBK Collective that analyzes the current state of Generative AI (Gen AI) adoption in enterprises.
The report explores how businesses are using Gen AI, the challenges they face, and the impact it has on their operations, employee roles, and investment strategies.
The report highlights key takeaways regarding the growing use of Gen AI across various functions, the increasing but slowing investment in the technology, and the evolving attitudes and perceptions of business leaders toward Gen AI.
It also provides insights into the leading Gen AI vendors and the most commonly used tools and platforms.
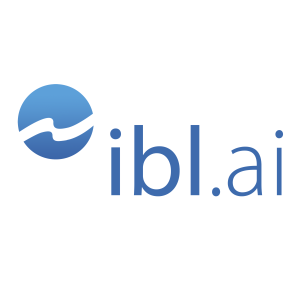
Friday Oct 18, 2024
Friday Oct 18, 2024
Summary of https://www.mckinsey.de/~/media/mckinsey/locations/europe%20and%20middle%20east/deutschland/news/presse/2024/2024%20-%2005%20-%2023%20mgi%20genai%20future%20of%20work/mgi%20report_a-new-future-of-work-the-race-to-deploy-ai.pdf
This report, written by the McKinsey Global Institute, examines the impact of artificial intelligence and automation on labor markets in Europe and the United States through 2030.
The report forecasts that certain jobs, particularly those requiring repetitive tasks, will be automated, resulting in a demand for workers with higher cognitive, technological, and social skills.
The report discusses potential job displacement and the need for workforce training programs to help workers transition to jobs in high demand.
The report also explores the impact of automation on productivity and the economic choices that companies and policymakers must make to manage the transition.