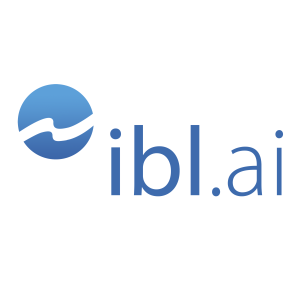
Monday Feb 10, 2025
University of Oxford: Who Should Develop Which AI Evaluations?
This research memo examines the optimal actors for developing AI model evaluations, considering conflicts of interest and expertise requirements. It proposes a taxonomy of four development approaches (government-led, government-contractor collaborations, third-party grants, and direct AI company development) and nine criteria for selecting developers.
The authors suggest a two-step sorting process to identify suitable developers and recommend measures for a market-based ecosystem fostering diverse, high-quality evaluations, emphasizing a balance between public accountability and private-sector efficiency.
The memo also explores challenges like information sensitivity, model access, and the blurred boundaries between evaluation development, execution, and interpretation. Finally, it proposes several strategies for creating a sustainable market for AI model evaluations.
The authors of this document are Lara Thurnherr, Robert Trager, Amin Oueslati, Christoph Winter, Cliodhna Ní Ghuidhir, Joe O'Brien, Jun Shern Chan, Lorenzo Pacchiardi, Anka Reuel, Merlin Stein, Oliver Guest, Oliver Sourbut, Renan Araujo, Seth Donoughe, and Yi Zeng.
Here are five of the most impressive takeaways from the document:
- A variety of actors could develop AI evaluations, including government bodies, academics, third-party organizations, and AI companies themselves. Each of these actors have different characteristics, and different strengths and weaknesses. The document outlines a framework for deciding which of these actors is best suited to develop specific AI evaluations, based on risk and method criteria.
- There are four main approaches to developing AI evaluations: AI Safety Institutes (AISIs) developing evaluations independently, AISIs collaborating with contracted experts, funding third parties for independent development, and AI companies developing their own evaluations. Each approach has its own advantages and disadvantages. For instance, while AI companies developing their own evaluations might be cost-effective and leverage their expertise, this approach may create a conflict of interest.
- Nine criteria can help determine who should develop specific evaluations. These criteria are divided into risk-related and method-related categories. Risk-related criteria include required risk-related skills and expertise, information sensitivity and security clearances, evaluation urgency, and risk prevention incentives. Method-related criteria include the level of model access required, evaluation development costs, required method-related skills and expertise, and verifiability and documentation.
- A market-based ecosystem for AI evaluations is crucial for long-term success. This ecosystem could be supported by measures such as developing and publishing tools, establishing standards and best practices, providing legal certainty and accreditation for third-party evaluators, brokering relationships between third parties and AI companies, and mandating information sharing on evaluation development. Public bodies could also offer funding and computational resources to academic researchers interested in developing evaluations.
- The decision of who develops AI evaluations is complex and depends on the specific context. The document emphasizes the importance of considering multiple factors, including the risk being assessed, the methods used, the capabilities of the potential developers, and the potential for conflicts of interest. It suggests that a systematic approach to decision-making can improve the overall quality and effectiveness of AI evaluations.
Comments (0)
To leave or reply to comments, please download free Podbean or
No Comments
To leave or reply to comments,
please download free Podbean App.