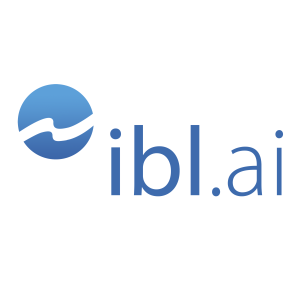
Wednesday Feb 05, 2025
University of Memphis: Generative AI in Education – From AutoTutor to the Socratic Playground
Summary of https://arxiv.org/pdf/2501.06682
This research paper investigates the potential of Large Language Models (LLMs) in revolutionizing education. The authors explore the parallels between LLMs and human cognition, examining both the opportunities and challenges of integrating generative AI into pedagogical practices.
They analyze the successes and limitations of earlier Intelligent Tutoring Systems (ITS), such as AutoTutor, before introducing the Socratic Playground, a next-generation ITS designed to overcome prior constraints. The paper emphasizes the importance of a pedagogy-first approach, ensuring that AI enhances—rather than overshadows—human teaching and learning.
Here are some interesting and non-mainstream takeaways from the sources:
-
Bidirectional Synergy Between LLMs and Human Cognition: The sources highlight a "bidirectional opportunity" where insights into Large Language Models (LLMs) can enhance our understanding of human cognition, and principles of human learning can guide the development of AI technologies. This suggests that studying AI can offer new perspectives on how humans learn, and vice versa, rather than being seen as completely separate fields. The NEOLAF (Never-Ending Open Learning Adaptive Framework) architecture exemplifies this synergy by integrating symbolic reasoning with neural learning.
-
Generative AI Exceeding Human Cognitive Performance: Generative AI, like the o3 model, has demonstrated the ability to exceed human cognitive performance in areas like mathematics and scientific problem-solving. This suggests AI is not just a tool for assisting humans, but has the potential to operate at a higher level of cognitive function in specific areas. This capability challenges the traditional view of AI as merely a helper or an automation tool.
-
Pedagogy-First Approach: The sources repeatedly emphasize that technology's success in education depends on its alignment with pedagogical principles. Simply integrating technology without careful thought about how it supports learning is unlikely to be effective. This suggests a shift from a technology-driven approach to a pedagogy-driven one, where educational goals guide the use of AI, rather than the other way around.
-
Limitations of Technology Alone: The sources make it clear that technology alone cannot replace the complex relational and motivational elements of teaching. This counters the narrative that AI could potentially replace human teachers altogether. The human element of teaching, including emotional intelligence and mentorship, remains critical.
-
Focus on Critical Thinking: The Socratic method is presented as a vital model for modern education, emphasizing critical thinking and inquiry over rote memorization. AI tools can enhance the Socratic method, but educators must still guide this process. This suggests that the goal of AI in education should be to cultivate critical thinking and deeper understanding, rather than simply automating tasks.
-
Beyond Limitations of LLMs: The sources note a shift in focus from the limitations of LLMs to harnessing their capabilities. This suggests a more optimistic and practical approach, emphasizing how advanced AI can enhance learning, rather than dwelling on its potential drawbacks.
-
Importance of Human Oversight: The sources repeatedly emphasize the importance of human oversight of AI tools, where teachers must learn how to operate AI tools but also how to scrutinize their outputs. This suggests that even the most advanced AI tools require careful human supervision to ensure that they are used effectively.
-
The Socratic Playground's Five Interactive Modes: The Socratic Playground for Learning offers five interactive modes (Assessment, Tutoring, Vicarious, Gaming, Teachable Agent) that are designed to personalize learning and encourage critical thinking. These modes provide a range of options that cater to different learning needs and preferences, which is a more nuanced approach than traditional one-size-fits-all instruction.
-
Teachable Agent Mode for Advanced Mastery: The "Teachable Agent Mode" in the Socratic Playground allows learners to teach a virtual student, solidifying their understanding. This approach leverages the principle that teaching enhances one's own learning and understanding. This mode suggests that the ultimate test of mastery is being able to explain the material to others, which is not a common approach in traditional learning settings.
-
JSON-Based Prompt Approach: The sources detail a JSON-based prompt approach that provides a structured way to guide AI tutors, ensuring transparency, modularity, and ease of maintenance. This illustrates how a systematic approach to prompt design can enhance the effectiveness of AI in education. This level of detail offers a behind-the-scenes view of how AI systems can be developed in a clear and transparent manner.
-
Learner's Characteristics Curve (LCC): The LCC, used within AutoTutor and applicable in the Socratic Playground, breaks down student responses into relevant/irrelevant and new/old components. This framework allows for fine-grained adaptivity and feedback, moving beyond simple right/wrong scoring to understand the nuances of a learner’s contributions.
-
Focus on Semantic Understanding: The prompt approach emphasizes semantic similarities and differences in student responses. This shows a move away from just matching keywords to actually understanding what a learner is trying to convey, which is a critical aspect of effective tutoring.
-
Iterative Learning Loops for Self-Improving Systems: Self-improving adaptive systems can refine their pedagogical logic based on large-scale learner data. These systems can simulate diverse virtual learners to predict the effectiveness of interventions, which can help educators adapt their teaching methods.
These takeaways highlight the complexities and potential of integrating AI into education, suggesting a more nuanced and thoughtful approach than simple automation.
Finally, the authors discuss future directions for AI in education, focusing on team tutoring, self-improving systems, and equitable access.
Comments (0)
To leave or reply to comments, please download free Podbean or
No Comments
To leave or reply to comments,
please download free Podbean App.