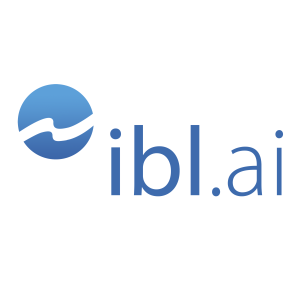
Monday Feb 10, 2025
University of Cambridge: Imagine While Reasoning in Space – Multimodal Visualization-of-Thought
Summary of https://arxiv.org/pdf/2501.07542
This research paper introduces Multimodal Visualization-of-Thought (MVoT), a novel approach to enhance complex reasoning in large language models (LLMs), particularly in spatial reasoning tasks.
Unlike traditional Chain-of-Thought prompting which relies solely on text, MVoT incorporates visual thinking by generating image visualizations of the reasoning process. The researchers implement MVoT using a multimodal LLM and introduce a token discrepancy loss to improve image quality.
Experiments across various spatial reasoning tasks demonstrate MVoT's superior performance and robustness compared to existing methods, showcasing the benefits of integrating visual and verbal reasoning. The findings highlight the potential of multimodal reasoning for improving LLM capabilities.
-
Multimodal Visualization-of-Thought (MVoT) is a novel reasoning paradigm that enables models to generate visual representations of their reasoning process, using both words and images. This approach is inspired by human cognition, which uses both verbal and non-verbal channels for information processing. MVoT aims to enhance reasoning quality and model interpretability by providing intuitive visual illustrations alongside textual representation.
-
MVoT outperforms traditional Chain-of-Thought (CoT) prompting in complex spatial reasoning tasks. While CoT relies solely on verbal thought, MVoT incorporates visual thought to visualize reasoning traces, making it more robust to environmental complexity. MVoT demonstrates better stability and robustness, especially in challenging scenarios where CoT tends to fail, such as in the FROZENLAKE task with complex environments.
-
Token discrepancy loss enhances the quality of generated visualizations. This loss bridges the gap between separately trained tokenizers in autoregressive Multimodal Large Language Models (MLLMs), improving visual coherence and fidelity. By minimizing the discrepancy between predicted and actual visual embeddings, it reduces redundant patterns and inaccuracies in generated images.
-
MVoT is more robust to environment complexity compared to CoT. CoT's performance deteriorates as environmental complexity increases, especially in tasks like FROZENLAKE, where CoT struggles with inaccurate coordinate descriptions. MVoT maintains stable performance across varying grid sizes and complexities by visualizing the reasoning process, offering a more direct and interpretable way to track the reasoning process.
-
MVoT can complement CoT and enhance overall performance. Combining predictions from MVoT and CoT results in significantly higher accuracy, indicating that they offer alternative reasoning strategies. MVoT can also be used as a plug-in for proprietary models like GPT-4o, improving its performance by providing visual thoughts during the reasoning process.
No comments yet. Be the first to say something!