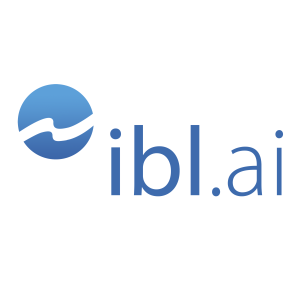
Monday Feb 17, 2025
University of California Irvine: What Large Language Models Know and What People Think They Know
This study investigates how well large language models (LLMs) communicate their uncertainty to users and how human perception aligns with the LLMs' actual confidence. The research identifies a "calibration gap" where users overestimate LLM accuracy, especially with default explanations.
Longer explanations increase user confidence without improving accuracy, indicating shallow processing. By tailoring explanations to reflect the LLM's internal confidence, the study demonstrates a reduction in both the calibration and discrimination gaps, leading to improved user perception of LLM reliability.
The study underscores the importance of transparent uncertainty communication for trustworthy AI-assisted decision-making, advocating for explanations aligned with model confidence.
The study examines how well large language models (LLMs) communicate uncertainty and how humans perceive the accuracy of LLM responses. It identifies gaps between LLM confidence and human confidence, and explores methods to improve user perception of LLM accuracy.
Here are 5 key takeaways:
- Calibration and Discrimination Gaps: There's a notable difference between an LLM's internal confidence in its answers and how confident humans are in those same answers. Humans often overestimate the accuracy of LLM responses, and are not good at distinguishing between correct and incorrect answers based on default explanations.
- Explanation Length Matters: Longer explanations from LLMs tend to increase user confidence, even if the added length doesn't actually improve the accuracy or informativeness of the answer.
- Uncertainty Language Influences Perception: Human confidence is strongly influenced by the type of uncertainty language used in LLM explanations. Low-confidence statements lead to lower human confidence, while high-confidence statements lead to higher human confidence.
- Tailoring Explanations Reduces Gaps: By adjusting LLM explanations to better reflect the model's internal confidence, the calibration and discrimination gaps can be narrowed. This improves user perception of LLM accuracy.
- Limited User Expertise: Participants in the study generally lacked the expertise to accurately assess LLM responses independently. Even when users altered the LLM's answer, their accuracy was lower than the LLM's.
No comments yet. Be the first to say something!