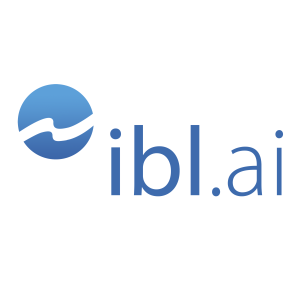
Friday Oct 18, 2024
Rice: Pedagogical Alignment of Large Language Models
Summary of https://arxiv.org/pdf/2402.05000
This research explores the use of Large Language Models (LLMs) as educational tools. The authors highlight the need to "pedagogically align" LLMs, meaning training them to provide structured, scaffolded guidance instead of direct answers.
The study proposes a novel approach using Learning from Human Preferences (LHP) algorithms, which leverage preference datasets to guide LLMs towards desired teaching behaviors. The research addresses the challenge of data scarcity by introducing a synthetic data generation technique using the CLASS framework.
Experiments with Llama, Mistral, and Zephyr models show that LHP methods significantly outperform standard supervised fine-tuning (SFT) in achieving pedagogical alignment.
The authors also introduce novel perplexity-based metrics to quantitatively measure the pedagogical alignment of LLMs.
Comments (0)
To leave or reply to comments, please download free Podbean or
No Comments
To leave or reply to comments,
please download free Podbean App.