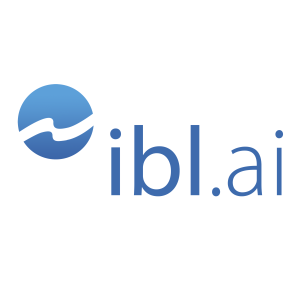
Thursday Apr 03, 2025
NIST: Adversarial Machine Learning – A Taxonomy and Terminology of Attacks and Mitigations
Summary of https://nvlpubs.nist.gov/nistpubs/ai/NIST.AI.100-2e2025.pdf
This NIST report explores the landscape of adversarial machine learning (AML), categorizing attacks and corresponding defenses for both traditional (predictive) and modern generative AI systems.
It establishes a taxonomy and terminology to create a common understanding of threats like data poisoning, evasion, privacy breaches, and prompt injection. The document also highlights key challenges and limitations in current AML research and mitigation strategies, emphasizing the trade-offs between security, accuracy, and other desirable AI characteristics. Ultimately, the report aims to inform standards and practices for managing the security risks associated with the rapidly evolving field of artificial intelligence.
-
This report establishes a taxonomy and defines terminology for the field of Adversarial Machine Learning (AML). The aim is to create a common language within the rapidly evolving AML landscape to inform future standards and practice guides for securing AI systems.
-
The report provides separate taxonomies for attacks targeting Predictive AI (PredAI) systems and Generative AI (GenAI) systems. These taxonomies categorize attacks based on attacker goals and objectives (availability breakdown, integrity violation, privacy compromise, and misuse enablement for GenAI), attacker capabilities, attacker knowledge, and the stages of the machine learning lifecycle.
-
The report describes various AML attack classes relevant to both PredAI and GenAI, including evasion, poisoning (data and model poisoning), privacy attacks (such as data reconstruction, membership inference, and model extraction), and GenAI-specific attacks like direct and indirect prompt injection, and supply chain attacks. For each attack class, the report discusses existing mitigation methods and their limitations.
-
The report identifies key challenges in the field of AML. These challenges include the inherent trade-offs between different attributes of trustworthy AI (e.g., accuracy and adversarial robustness), theoretical limitations on achieving perfect adversarial robustness, and the complexities of evaluating the effectiveness of mitigations across the diverse and evolving AML landscape. Factors like the scale of AI models, supply chain vulnerabilities, and multimodal capabilities further complicate these challenges.
-
Managing the security of AI systems requires a comprehensive approach that combines AML-specific mitigations with established cybersecurity best practices. Understanding the relationship between these fields and identifying any unique security considerations for AI that fall outside their scope is crucial for organizations seeking to secure their AI deployments.
No comments yet. Be the first to say something!