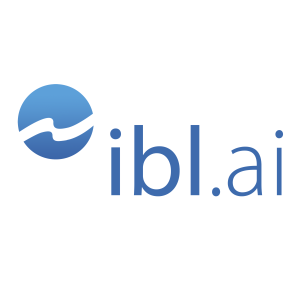
Thursday Mar 20, 2025
CSET: Putting Explainable AI to the Test – A Critical Look at Evaluation Approaches
This Center for Security and Emerging Technology issue brief examines how researchers evaluate explainability and interpretability in AI-enabled recommendation systems. The authors' literature review reveals inconsistencies in defining these terms and a primary focus on assessing system correctness (building systems right) over system effectiveness (building the right systems for users).
They identified five common evaluation approaches used by researchers, noting a strong preference for case studies and comparative evaluations. Ultimately, the brief suggests that without clearer standards and expertise in evaluating AI safety, policies promoting explainable AI may fall short of their intended impact.
- Researchers do not clearly differentiate between explainability and interpretability when describing these concepts in the context of AI-enabled recommendation systems. The descriptions of these principles in research papers often use a combination of similar themes. This lack of consistent definition can lead to confusion and inconsistent application of these principles.
- The study identified five common evaluation approaches used by researchers for explainability claims: case studies, comparative evaluations, parameter tuning, surveys, and operational evaluations. These approaches can assess either system correctness (whether the system is built according to specifications) or system effectiveness (whether the system works as intended in the real world).
- Research papers show a strong preference for evaluations of system correctness over evaluations of system effectiveness. Case studies, comparative evaluations, and parameter tuning, which are primarily focused on testing system correctness, were the most common approaches. In contrast, surveys and operational evaluations, which aim to test system effectiveness, were less prevalent.
- Researchers adopt various descriptive approaches for explainability, which can be categorized into descriptions that rely on other principles (like transparency), focus on technical implementation, state the purpose as providing a rationale for recommendations, or articulate the intended outcomes of explainable systems.
- The findings suggest that policies for implementing or evaluating explainable AI may not be effective without clear standards and expert guidance. Policymakers are advised to invest in standards for AI safety evaluations and develop a workforce capable of assessing the efficacy of these evaluations in different contexts to ensure reported evaluations provide meaningful information.
No comments yet. Be the first to say something!